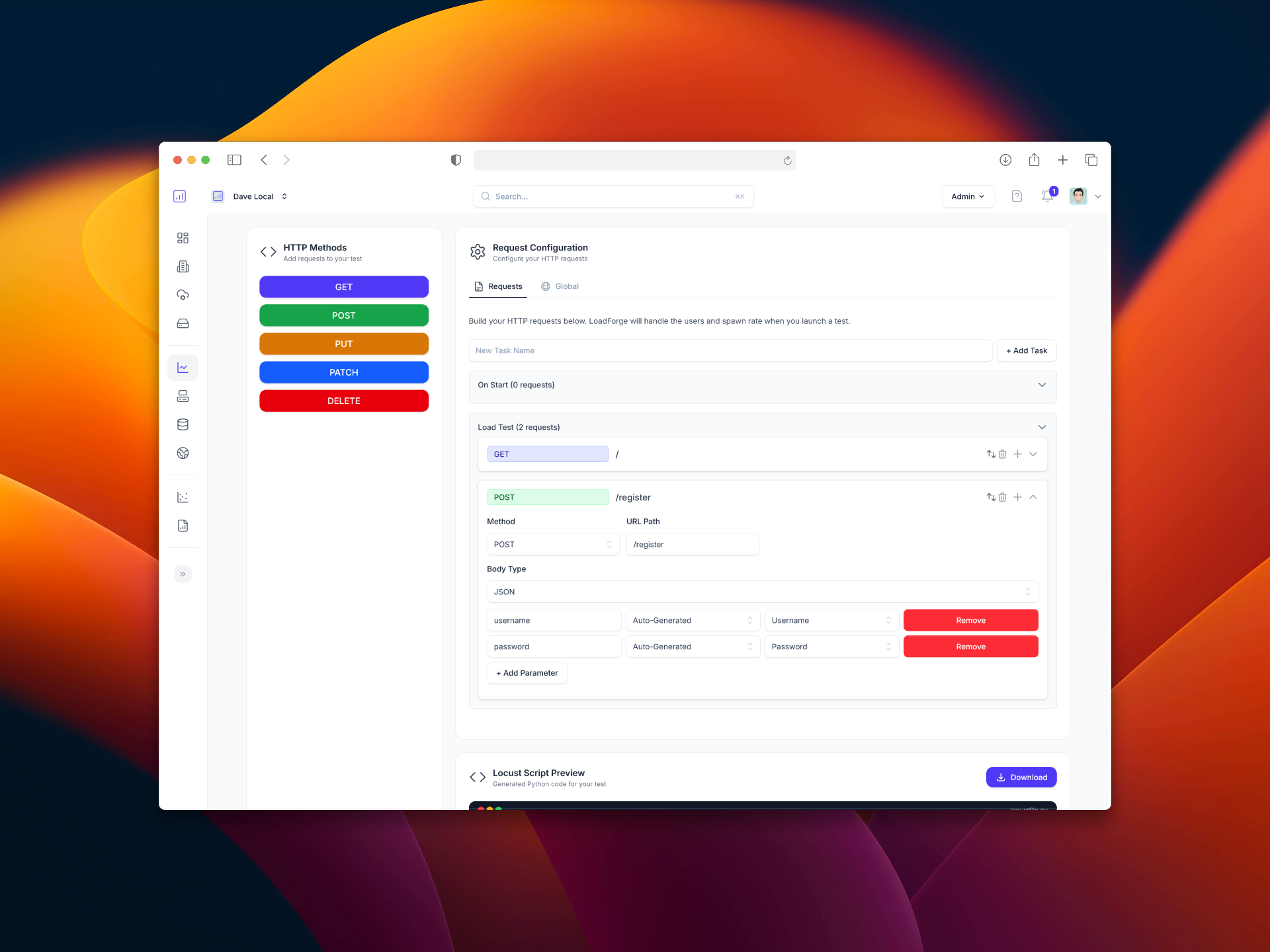
Jira Integration & Graphical Test Builder
We are proud to announce the release of two new features, available immediately. 🚀 Jira Integration: Automate Issue Tracking for...
When conducting load testing, one of the most vital scenarios to simulate is user logins. This process is crucial because it typically represents a user's first interaction with your application and can heavily influence their overall experience and impression of...
When conducting load testing, one of the most vital scenarios to simulate is user logins. This process is crucial because it typically represents a user's first interaction with your application and can heavily influence their overall experience and impression of your service. By accurately simulating user logins during load testing, you can gain valuable insights into how your system behaves under stress from multiple concurrent users trying to access their accounts.
Simulating user logins in load tests is essential for several reasons:
Realism: Real-world users log in to access personalized or restricted content. Testing without simulating these logins can lead to overlooking potential bottlenecks and issues that only arise during the authentication process.
Performance Impact: Login requests often involve database queries, session starts, and other resource-intensive operations. Understanding their impact on system performance is necessary for scaling and optimization.
Security and Reliability: Properly testing the login feature ensures that your application can handle high traffic while maintaining security standards, preventing unauthorized access and safeguarding user data even under pressure.
User Experience (UX) Optimization: By analyzing how long it takes a user to log in during high traffic times, you can make necessary adjustments to improve user satisfaction and decrease abandonment rates.
Simulating user logins in a load test involves generating multiple virtual users that perform login operations, as they would in a live environment. These users will typically:
This integral part of testing is not just about hammering the login form with requests but rather ensuring that every part of the process from the initial request to the final user landing page behaves as expected under various conditions and loads.
Including user login simulations in your load testing strategy is indispensable for crafting a resilient, user-centric, and high-performing application. Continuating onto understanding the user login process, setting up testing environments, and crafting the actual Locustfiles will further solidify your load testing approach, reinforcing the importance of rigorous, real-world simulation conditions.
Before you can effectively simulate user logins during load testing, it's crucial to have a comprehensive understanding of the login process and the common elements involved. This section will cover the key components such as HTTP requests, session management, and data handling. Each of these plays a significant role in how user logins are simulated and how accurately they reflect real-world user behavior on your application.
User logins typically involve several HTTP requests. Here's a typical flow:
GET Request to Login Page: A user initially makes a GET request to retrieve the login page. This often includes static resources like CSS and JavaScript files necessary for rendering the page.
GET /login
POST Request for Authentication: Upon submitting login credentials, your application usually handles this with a POST request. This request sends user details (username and password) to the server for authentication.
POST /login
{
"username": "user",
"password": "pass"
}
After a successful login, session management becomes key:
Session Creation: The server creates a session which serves as an agreement between the user and the server that they are who they say they are. This session is usually identified by a session ID.
Cookie/Token Handling: The server sends a cookie or token back to the client. This token or cookie is used to maintain the session on subsequent requests.
response.set_cookie('session_id', new_session_id)
Session Store: Sessions are often stored in server-side databases or memory, allowing users to make further secured requests without re-authentication.
Effective simulation of logins also requires understanding how user data is handled:
Credentials Storage: How the application stores and retrieves user credentials (hopefully securely, e.g., hashed passwords in databases).
Input Validation: Ensures the inputs during login are handled correctly, preventing common vulnerabilities like SQL Injections.
import sqlite3
# This is a simplistic and insecure example:
conn = sqlite3.connect('users.db')
c = conn.cursor()
c.execute(f"SELECT * FROM users WHERE username='{username}' AND password='{password}'")
Error Handling: Appropriate error messages should be returned to the user for issues like incorrect passwords or user not found, without revealing too much about the database or structure.
These components—HTTP requests, data handling, and session management—are crucial in replicating a realistic login process during load testing. Accurately simulating this process enables more reliable and meaningful test results, providing insights into how your application will perform under various load conditions.
Preparing your LoadForge environment for simulating user logins involves several crucial steps. This section provides guidance on how to configure your LoadForge settings, choose the right test regions, and outline potential user scenarios. By following these configurations, you'll ensure your test environment accurately reflects real-world usage, and captures essential metrics that inform the performance and scalability of your application’s login system.
Before running any tests, configure your LoadForge setup to match the expected production environment as closely as possible. This includes setting up:
Virtual Users: Determine how many virtual users you will simulate logging in simultaneously. This figure should reflect your anticipated maximum user load during peak times.
Test Duration: Set the duration of the test. This should be long enough to observe how the system behaves under sustained load.
Network Conditions: Simulate various network speeds and latency to understand how your application’s login performs under different conditions.
Use the LoadForge dashboard to easily adjust these settings:
# Example Configuration
{
"users": 5000,
"spawn_rate": 150,
"time": "10m",
"network": "3G"
}
Choosing the right test regions is critical for obtaining meaningful results, especially for geographically dispersed user bases. LoadForge allows tests to be run from multiple locations around the world, which provides insights into how geographical distribution impacts the login performance.
Consider the following when selecting your test regions:
User scenarios help simulate real-world interactions with your application. When configuring user logins, consider different use cases:
Example template for a basic login scenario in a Locustfile script:
from locust import HttpUser, task, between
class WebsiteUser(HttpUser):
wait_time = between(1, 3)
@task
def login(self):
self.client.post("/login", {"username":"testuser", "password":"secret"})
Remember, the accuracy of your test results heavily depends on how well you can mimic real user behavior. Take your time to detail each scenario, ensuring that they mirror actual user interactions as closely as possible.
Setting up your test environment is a foundational step in ensuring your load testing provides meaningful, actionable data. By carefully configuring your LoadForge environment, selecting appropriate test regions, and crafting detailed user scenarios, you're well on your way to a successful simulation of user logins. This setup not only helps identify potential bottlenecks but also enhances overall system resilience and performance.
In this core section of our guide, we will dive into how to construct a Locustfile script specifically designed to simulate multiple users logging into your system. This step-by-step guide will provide detailed code examples to help you set up your load testing configuration accurately.
Start by importing the required modules in your Locustfile script. The primary module needed is locust
, but you'll also often use HttpUser
, task
, and between
from the locust library.
from locust import HttpUser, task, between
Create a class that represents a user behavior. This class will inherit from HttpUser
, which provides each user with HTTP capabilities. Use the task
decorator to define the behavior (i.e., logging in).
class WebsiteUser(HttpUser):
wait_time = between(1, 5)
@task
def login(self):
self.client.post("/login", {"username": "test_user", "password": "PASSWORD"})
Handling sessions correctly is crucial to simulate a user that remains logged in. The self.client
automatically manages cookies, which in turn handle the sessions. Here's how you'd typically handle a login request:
@task
def login(self):
with self.client.post("/login", {"username": "test_user", "password": "password"}, catch_response=True) as response:
if response.status_code == 200 and "authentication token" in response.text:
self.token = response.json()['token']
else:
response.failure("Failed to log in")
To simulate multiple users, you can adjust the min_wait
and max_wait
attributes of the HttpUser class, or use the between()
function as shown earlier. These settings define the time that simulated users wait between executing tasks.
After logging in, you might want users to perform additional tasks. Extend the WebsiteUser
class with more @task
methods:
@task(3)
def view_profile(self):
self.client.get("/profile", headers={"Authorization": f"Bearer {self.token}"})
@task(1)
def log_out(self):
self.client.post("/logout", headers={"Authorization": f"Bearer {self.token}"})
Here, @task(3)
means the view_profile
task is 3 times more likely to be picked than log_out
.
Below your user class, configure the load test to simulate more realistic scenarios by adjusting the number of users and the spawn rate:
from locust import HttpUser, task, between
class WebsiteUser(HttpUser):
# [Insert user tasks here as defined above]
# Specify the number of simulated users and spawn rate
class MyBaseLoadTest(HttpUser):
host = "https://yourwebsite.com"
tasks = [WebsiteUser]
min_wait = 5000
max_wait = 15000
By following these detailed steps, you have successfully written a basic Locustfile script to simulate multiple users logging into a system concurrently. This script forms the foundation of your load testing strategy, allowing you to adjust scenarios, user behaviors, and configurations to meet your testing requirements.
Executing your load test on LoadForge is a critical step in determining whether your application can handle the stress of multiple users logging in simultaneously. In this section, we will guide you through the process of running the test, monitoring it in real-time, and interpreting the initial results to ensure your testing setup is configured correctly.
Upload Your Locustfile: Begin by logging into your LoadForge account. Navigate to the "Scripts" section and upload your Locustfile script that you have prepared to simulate user logins. Ensure the script is free from errors by reviewing it closely before uploading.
Create a New Test:
Set Test Parameters: Choose the duration for which the test should run and the region(s) from which the test should be initiated. Make sure to select regions closest to your user base to get the most accurate results.
Start the Test: After setting all parameters, click on the “Start Test” button to initiate the testing process. The platform will begin to deploy virtual users as per the configurations set.
Once the test is running, you can monitor its progress in real-time:
Dashboard Overview: LoadForge provides a detailed dashboard that shows various metrics such as total requests, response times, number of users, and failures. This live data is crucial for spotting any immediate issues.
Response Times and Failures: Keep an eye on the average, median, and 95th percentile response times. Also, watch for any increasing failure rates which might indicate issues like login failures or server errors.
Resource Utilization: Monitor CPU and memory usage on your server if possible. High utilization may reveal bottlenecks in your system's capacity to handle concurrent logins.
After the completion of the test, LoadForge will provide a summary of the test execution, which includes:
Total and Failed Requests: Review the number of successful and failed login attempts. A high failure rate could be indicative of issues like incorrect login logic or database bottlenecks.
Performance Graphs: Analyze the response time graphs and error rate graphs. Look for any spikes or anomalies that correspond with high loads.
Comparison with Previous Tests: If this isn’t the first test, compare the outcomes with previous runs to determine if changes or improvements in the code or configuration have had the desired effect.
Logs and Errors: Inspect the logs for any errors or exceptions that were captured during the test. These logs can provide detailed insights into what might be going wrong.
Example of a typical error log analysis:
[ERROR] Login failed for user user@example.com: Response code 500, expected 200.
Understanding these elements after executing your test on LoadForge will aid you in ensuring that your setup is accurately configured and capable of handling the intended load. In the event of undesirable results or errors, revisit your script configuration or server setup before running additional tests.
After successfully executing your login simulation tests on LoadForge, the next crucial step is to analyze the results. This analysis helps you identify any potential bottlenecks and understand how user logins impact the overall performance of your application. This section will guide you through the process of analyzing your test data, interpreting the key metrics, and using this information to refine your test for better outcomes.
Initially, log in to your LoadForge dashboard to access the results of your test. LoadForge provides a comprehensive set of metrics displayed in graphs and tables, which include:
Review these metrics to get an initial sense of how well your application managed the simulated login load.
Look for any anomalies or spikes in response times and error rates. Significant increases typically indicate a bottleneck. Common areas to examine include:
Once you've spotted potential issues, drill down for more details. If LoadForge reports high error rates during peak load times, examine server logs and error messages to identify the root causes. For database issues, utilize query logs to see which queries are taking longer to execute and consider optimizing them or improving indexing.
Compare the current test results with previous runs or baseline metrics to determine if the performance is improving or degrading over time. This comparison can highlight the impact of recent code or infrastructure changes.
For deeper analysis, consider exporting the raw data from LoadForge to a tool like Excel, Google Sheets, or a more sophisticated analytics platform like Grafana. This allows for further customization of data visualization and more detailed performance trend analysis.
# Example of exporting CSV data from LoadForge
curl -o test_results.csv 'https://api.loadforge.com/tests/123/results/csv/'
Consolidate your findings in a document and share them with your development and QA teams. Based on the insights gained:
As a best practice, set up continuous monitoring of your login process performance. Integrating LoadForge with monitoring tools can help you automatically track and respond to performance issues in real time.
By thoroughly analyzing the test results from your simulated user logins and continually iterating on your testing strategies, you ensure your application can handle real-world usage scenarios efficiently and effectively. This process not only helps in identifying weaknesses but also in building a robust framework capable of supporting user growth and peak load conditions.
During the process of load testing user logins, several common issues might arise. These problems can skew results, lead to misinterpretations of performance capabilities, or even crash the testing environment. Here, we discuss these issues and offer practical solutions to ensure that testing is both efficient and reflective of actual user behavior.
Problem: The number of virtual users (VUs) is too low to effectively stress test the login system.
Solution: Increase the number of VUs within your Locustfile. Consider gradually scaling up the VU count to understand at what point your system begins to falter.
from locust import HttpUser, task, between
class WebsiteUser(HttpUser):
wait_time = between(1, 5)
@task
def login(self):
self.client.post("/login", {"username":"foo", "password":"bar"})
Problem: Form validation errors due to incorrect or inconsistent input data in the test scripts.
Solution: Ensure all form fields are correctly populated. Use realistic data sets and consider using variables from a CSV file or a list to simulate different user inputs dynamically.
from locust import HttpUser, task, between
import csv
class WebsiteUser(HttpUser):
wait_time = between(1, 5)
@task
def login(self):
with open('user_credentials.csv', 'r') as file:
reader = csv.reader(file)
for row in reader:
self.client.post("/login", {"username": row[0], "password": row[1]})
Problem: Login requests time out or fail due to network congestion or server unavailability.
Solution: Review server logs to locate bottlenecks. If timeouts are frequent, increase timeout settings in your Locust configuration and possibly enhance server capacity or optimize server response time.
class WebsiteUser(HttpUser):
# Increase the connection timeout
def __init__(self):
super().__init__()
self.client.timeout = 30 # Timeout set to 30 seconds
Problem: Sessions might not be maintained across tasks, resulting in failed login attempts or dropped sessions.
Solution: Use Locust's on_start
method to login users and maintain session cookies across subsequent tasks.
from locust import HttpUser, task, between
class WebsiteUser(HttpUser):
wait_time = between(1, 5)
def on_start(self):
self.client.post("/login", {"username": "foo", "password": "bar"})
@task
def indexed_page(self):
self.client.get("/my_profile")
Problem: Tests are unintentionally triggering rate limits or firewall rules, which can block testing IPs.
Solution: Adjust the rate of requests in your locust tasks or coordinate with your IT/security team to whitelist test IPs during load tests.
class WebsiteUser(HttpUser):
wait_time = between(1, 3) # Slower rate of requests
Problem: Not accounting for various HTTP response codes can lead to misleading test outcomes.
Solution: Implement response checks in your Locust tasks and log different scenarios to fully understand the effects of various loads.
@task
def login(self):
with self.client.post("/login", {"username": "foo", "password": "bar"}, catch_response=True) as response:
if response.status_code == 200:
response.success()
else:
response.failure("Failed to login")
Properly addressing these issues will make your load tests more reliable and informative, enabling you to make better-informed decisions on system scalability and performance.
Simulating user logins during load testing is a complex process that requires careful planning, execution, and analysis to ensure your application can handle real-world usage scenarios efficiently, reliably, and without disruption. Following these best practices will help create a robust simulation environment and drive valuable, actionable insights from your load testing efforts.
Ensure that the user credentials and data used in the test are as realistic as possible. Avoid using the same credentials for all virtual users; instead, use a diverse set of credentials that mimic real user behavior.
from locust import HttpUser, task, between
class WebsiteUser(HttpUser):
wait_time = between(1, 5)
def on_start(self):
self.login()
def login(self):
response = self.client.post("/login", {
"username": "user" + str(self.environment.runner.get_current_user_count()),
"password": "password"
})
self.token = response.json()['token']
Properly manage sessions in your tests to reflect the real-world user experience. Make sure cookies or authentication tokens are correctly handled after each login attempt to simulate continuous authenticated sessions accurately.
Even if your purpose is to simulate high traffic, it's essential to introduce delays or think time between login requests to avoid unrealistic load conditions that could lead to skewed results.
Include a variety of login scenarios in your tests. For example, simulate conditions where login attempts fail due to incorrect credentials or other reasons to see how your system manages these exceptions during high traffic.
Focus on critical performance metrics such as response time, error rates, and concurrent sessions during and after the test. Tools like LoadForge's dashboard can provide real-time monitoring and detailed post-test analysis to help understand the impact of user logins under load.
Always ensure proper cleanup after tests, especially in production-like environments. Clear out session data, tokens, and other temporary data created during tests to maintain system integrity and security.
Prepare for different network conditions and latencies, especially if your application caters to a global audience. Testing from various regions using LoadForge can help identify region-specific performance issues.
Use the insights gained from each test to refine your testing strategies and simulation scripts. Continuous improvement will help catch issues early and adapt to changing user behaviors and application updates.
Following these best practices will help ensure that your simulated user logins provide a realistic gauge of how well your web application can handle the demands of actual users in production. Proper planning, execution, and repeated testing are key to maintaining performance and enhancing user satisfaction.